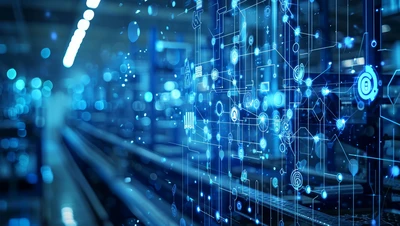
OEE's dark little secret
Dec 2, 2024
Why relying on overall equipment effectiveness alone is failing your factory and what to do about it
If you work in manufacturing, chances are that one of the Key Performance Indicators (KPIs) your factory relies on to optimize production is Overall Equipment Effectiveness (OEE). This useful metric provides a snapshot of machine performance and is often used as a proxy to measure overall production efficiency. It combines availability, performance, and quality into a single percentage-based number.
However, if you’ve spent significant time on the shop floor or in manufacturing management, you are likely to know the practical limitations of OEE. By focusing solely on equipment utilization, traditional OEE systems ignore human factors, bottlenecks, supply chain delays, and process inefficiencies. OEE is also frequently used to assess processes it was not designed for, leading to misleading conclusions and missed opportunities for improvement. Without considering these broader issues, OEE provides an incomplete picture of production performance. Here, I discuss why OEE might not be a magic bullet, and how modern software-based approaches can effectively overcome its limitations.
Common problems with traditional OEE softwares
OEE in manufacturing: How industry typically calculates OEE
The OEE is a key figure that measures the productivity of a machine or production line. To calculate the overall equipment effectiveness, the three main components are availability, production efficiency and quality rates. These are normally consolidated into a single number using the formula:
OEE = Availability x Production efficiency x Quality rate
It’s just a number, not the whole story
OEE oversimplifies operations, providing a high-level view that can mask real problems. A good OEE score doesn’t necessarily mean the process is trouble-free and could be hiding issues like product-related quality issues or underlying process inefficiencies.
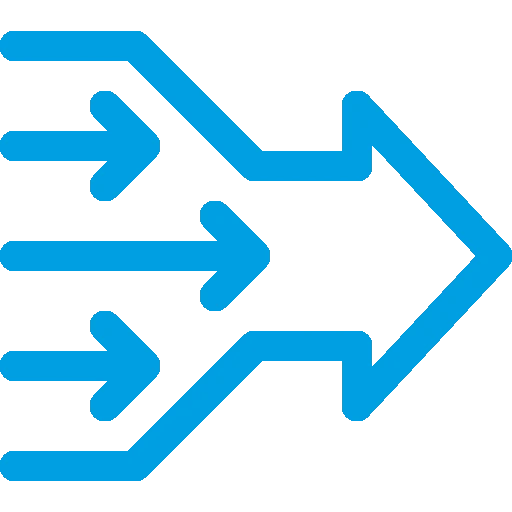
OEE can actually harm overall efficiencies
Maximizing OEE can paradoxically slow down the system, by prioritizing resource utilization above flow efficiency. OEE focuses solely on machine performance without considering the efficiency of work flowing through the entire process. Optimizing individual machines can reduce overall system efficiency, exemplifying the “efficiency paradox.”*
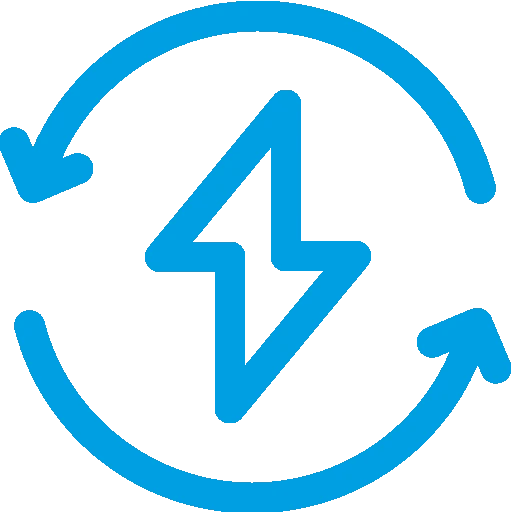
Accurate data? Only in theory
While the calculation for OEE is considered precise, its data collection process is often inconsistent and prone to human error or manipulation. Various personnel shifts or staff may interpret events differently, resulting in unreliable data and misleading scores. Adjustments for localized conditions reduce transparency further, making it hard to compare performance across machines, production lines, or sites.
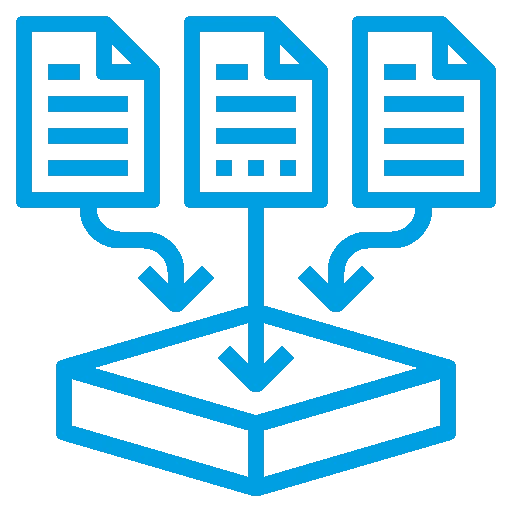
Manual inputs only as good as management’s discipline
Manual data entry is error-prone and inconsistent and requires a lot of managerial discipline to ensure good-quality data. Better machine integration can standardize inputs but is costly and does not scale well in facilities with diverse hardware. Consequently, many systems still use manual inputs, which are time-consuming, unreliable and expensive.
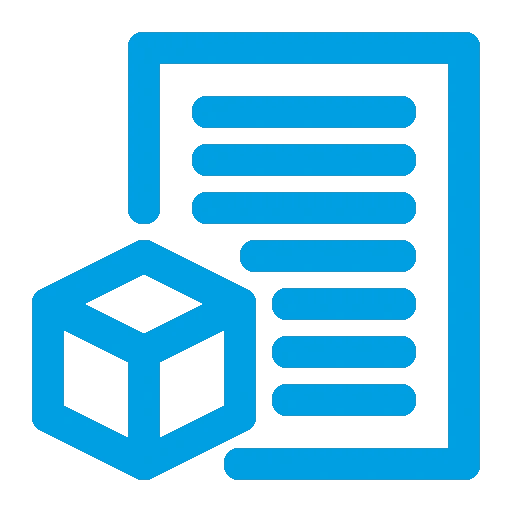
High costs of integration and maintenance
Setting up and maintaining a system to measure production efficiency and improve OEE can be costly and tedious. It often requires integrating machines with data systems (like ERP, MES, and APS) and heavy investment in sensors, connectivity, and software. Mounting costs can mean that smaller operations or less critical steps are excluded. Maintaining these systems also demands dedicated personnel, which further increases operational expenses.
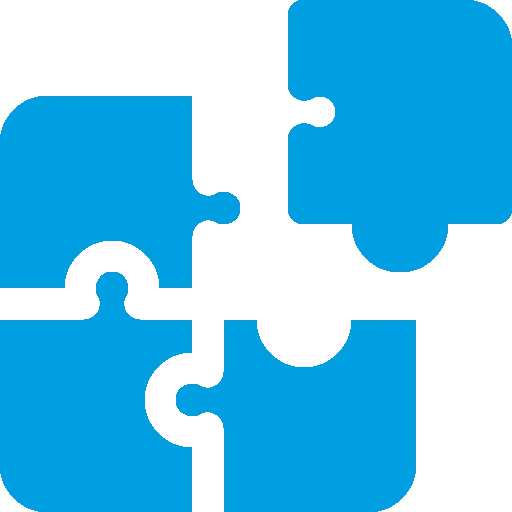
Reactive, not proactive
Traditional OEE systems are primarily retrospective, analyzing problems after they occur. This leaves little scope for preventative action, as operators can address issues only after they escalate and cause unnecessary downtime or inefficiencies.
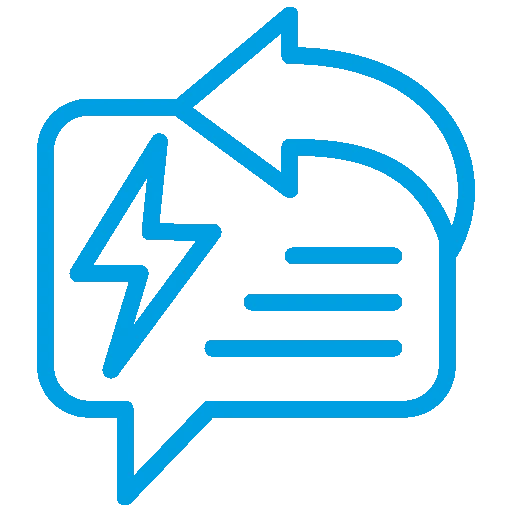
Shortcuts over solutions
Operators may even push to boost OEE scores at the expense of proper maintenance or sustainable practices. While short-term gains might look impressive, they can lead to long-term breakdowns and higher costs.
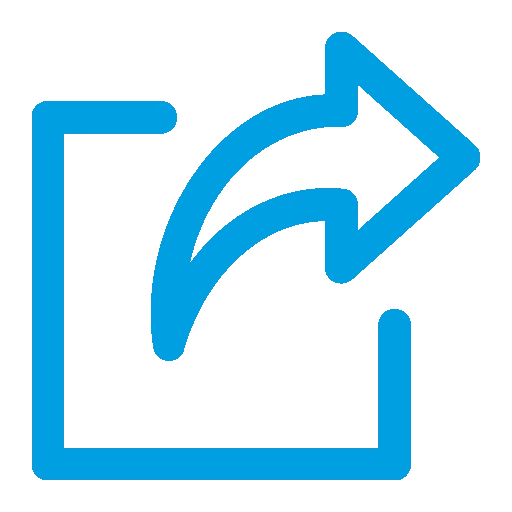
Ignores the human element
Traditional OEE systems fail to capture the insights and expertise of human operators. This omission hinders continuous improvement and ignores valuable knowledge, especially in environments with high turnover rates and scarce skilled labor.
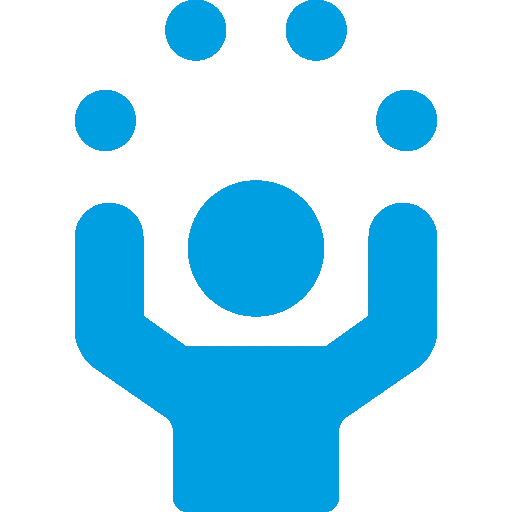
The 21st Century Approach to OEE
Given these challenges, it’s worth asking whether the traditional approach to OEE is still practical for improving overall production efficiency in today’s connected world. Modern software tools based on Artificial Intelligence (AI) and Machine Learning (ML) allow us to define new paradigms for resource optimization. These tools are easier to use and maintain, provide more flexibility to measure and contextualize data, and offer a holistic approach to improving manufacturing facilities while reducing operational complexities. Below, I take the example of the iTAC.Asset.Analyzer to demonstrate this modern approach to OEE.
Streamlining your connectivity conundrum
Unlike traditional OEE systems, modern software tools use AI and ML methods to infer system parameters directly from machine data streams. The iTAC.Asset.Analyzer automatically analyzes historical data to deduce factors like shift utilization, product changeovers, and unscheduled breakdowns and does not need extensive configuration or manual intervention for each product type.
Simplified connectivity makes these systems faster and cheaper to implement, delivering actionable insights much sooner than traditional Business Intelligence and OEE systems. Addressing the “connectivity conundrum”* which manufacturers face, this modern approach:
- Avoids costly integration with MES, ERP, and planning systems.
- Reduces errors and inconsistencies arising from manual configuration.
- Minimizes the need for human intervention to maintain data sources.
- Lowers the risk of results manipulation.
Figure 1: Normally a substantial amount of additional data is required to calculate OEE using the traditional calculation methodologies, resulting in costly integration efforts with a factory’s ERP and Planning Tool to automate. Above, we can see an example of how state-of-the-art tools can intelligently infer key parameters from existing machine data, without the need to integrate multiple systems. In the top diagram, we can see how the system automatically identified planed productive time (PT) and non-productive time (NPT). In the bottom diagram, we can see where AI has automatically recognised change overs on the line, with each product illustrated in a different colour. This also has the added benefit that it eliminates a lot of human intervention and unnecessary manual data entries, reducing the possibility of human error.
Adopting real-time monitoring and workflow triggers
Modern tools offer real-time analytics, enabling immediate responses to issues. The iTAC.Asset.Analyzer notifies teams of potential problems as they arise, thereby preventing downtime and improving overall efficiency. Operations thereby move from reactive to proactive and alarms, errors or outlier measurements can be used to trigger automated responses.
Figure 2: The iTAC.Asset.Analyzer’s system automatically detects anomalous system behaviour by monitoring measurement data and detecting outliers in the data set in real time. These can be used to set alarms or trigger workflows if certain thresholds are reached.
Figure 3: An example of an automated message sent by the iTAC.Asset.Analyzer to the process engineering team on shift duty to investigate a problem at the Conformal Coating Inspection (CCI) machine after it identified an unusually high cluster of quality problems. Here an external workflow is triggered, whereby the team is informed of anomalous behaviour and impeding situation via MS Teams.
Leveraging digital twins technology
AI-powered virtual models simulate production lines, analyzing how machines interact under changing conditions. The iTAC.Asset.Analyzer’s Downtime Tracker identifies bottlenecks as well as upstream or downstream issues, and their impact on overall line performance. This holistic view encourages better decision-making and more effective resource utilization.
Figure 4: Modern tools understand not only the dynamic of each machine, but also how the behaviour of all machines on the line are affected by each other in certain situations like breakdowns. Above we see how the iTAC.Asset.Analyzer uses digital twin models to augment the real-time data stream and provide very accurate state representatives of each machine and the overall status of the line. These give production staff a deeper understanding of the root causes behind production outages and reduced output.
Figure 5: The iTAC.Asset.Analyzer’s real-time tracking uses an efficiency matrix to show system users how efficiently individual resources in a production line are utilized, and how much they contribute to total flow efficiency. The Perfect State is machines running both efficiently and lean, shown in the top left corner of the matrix. Islands of Efficiency, or machines running efficiently in isolation but not in relation to other machines in the line, are shown in the top right corner. Efficient Oceans, or machines running synchronously with each other but being underutilized, are in the bottom right corner. Finally, machines in the Wasteland category (bottom left corner) are not resource or flow efficient and make up the most undesirable scenario. In this example we see that the bottleneck machine (pnp_2) is running below maximum resource efficiency. This situation is not ideal, and management can immediately recognize that there are huge potential productivity gains to be made across the entire line if the utilization of this one machine can be improved.
Integrating human insights
An innovative system like the iTAC.Asset.Analyzer’s AI Playbook captures human expertise, documents human actions to identify their root causes and even provides actionable recommendations. This helps teams learn from past challenges, develop proactive solutions, and continuously improve their performance. Fully integrated Connected Worker Solutions such as these are empowering next-generation AIs to deliver unprecedented efficiency gains.
Figure 6: An example menu from the iTAC.Asset.Analyzer that allows operators to add additional comments information during the problem resolution process, allowing their process knowledge and factory expertise to merge with real-time data streams.
The bottom line
While OEE is a useful metric, it’s not the ultimate solution for improving efficiency in modern manufacturing. Today’s factories require tools that go beyond machine performance to consider flow efficiency, real-time monitoring, and human expertise. AI-driven systems provide a more practical and effective approach, combining data, context, and proactive strategies to drive meaningful change on the shop floor. True productivity isn’t about chasing high OEE scores—it’s about achieving sustainable improvements through smarter, integrated solutions.
*Further reading
Liker, J.K., 2004. The Toyota way; The company that invented lean production.
Modig, N. and Åhlström, P., 2012. This is lean: Resolving the efficiency paradox. Vol. 41. Stockholm: Rheologica.
Riggs, J.L., 1987. Production systems: planning, analysis, and control. Vol. 582. New York: Wiley.
Toro, C., Wang, W. and Akhtar, H., 2021. Implementing Industry 4.0. Springer International Publishing.
Womack, J.P., Jones, D.T. and Roos, D., 2007. The machine that changed the world: The story of lean production--Toyota's secret weapon in the global car wars that is now revolutionizing world industry. Simon and Schuster.